Reviewing the Role of Artificial Intelligence in Cancer
Download
Abstract
Among the modern-day advancements in technology, artificial intelligence (AI) and machine learning have shown to possess a large number of applications in various fields including medicine. In the current era, cancer is one of the most common non-communicable diseases attributing to a large number of global deaths. Attempts to increase the mortality and morbidity of cancer has been ongoing research. The integration of Artificial intelligence into cancer research is being actively carried out and has provided very promising results. Major factors that play a vital role in improving cancer prognosis including early detection and accurate diagnosis employing various imaging and molecular techniques. The use of artificial intelligence as a tool in these areas has shown its potential to detect and diagnose with increased precision, which is just one of the many applications of AI in cancer research. The present review aims as delving into literature and enlisting the applications of artificial intelligence in various commonly occurring cancers.
Introduction
The human body comprises trillions of cells, and the chance of occurrence of cancer in any part of it is fairly significant. Non-communicable diseases (NCDs) are now a major cause of global deaths, and cancer is expected to top the ranked list of leading causes of deaths in every country [1]. Cancer incidences and mortality are rapidly growing worldwide [2]. As per the report of the World Health Organization (WHO) in 2015, cancer is one of the topmost ranking causes of death before the age group of 70-75 years in 91 countries out of 172 and holds the third or possibly fourth position in 22 other countries.
One of the major factors that play a vital role in tackling cancer is its early detection and prompt diagnosis. There are different imaging techniques available for cancer screening and diagnosis among which the investigative methods that top the list are mammography, ultrasound, and thermography. Mammography is one of the most important early diagnostic methods for breast cancer but it is not very successful for dense breasts. For this reason, ultrasound or diagnostic sonographic techniques are recommended [3]. In recent years, technological advancement in medical imaging as well as the discovery of minimally invasive biomarkers have shown possibilities of curbing such challenges across a wide spectrum including detection of cancer, therapeutics and monitoring techniques. However, one of the major challenges lies in the interpretation of the large volume of data being generated by such advancements.
Over the past few years, Machine Learning’s (ML’s) potential in precision oncology has become more apparent. The application of deep learning (DL), a broader part of ML, in wide array of aspects including diagnosis, prognostic determination, and prediction tasks have been reported [4-7]. DL has shown an impressive performance in the classification of image data in varied clinical fields. Advances in DL has greatly improved its efficiency and precision in oncology. Few examples would include detecting and classifying skin lesions, the identifying and categorizing of lung cancers, detecting breast cancer metastases and the like. All these DL techniques on images primarily employ convolutional neural networks (CNNs) [8-11]. Different Machine learning approaches have been utilized in the field of oncology. These include analysis of datasets from varied sources using both supervised and unsupervised learning [12-13].
Concepts of Artificial Intelligence, Machine Learning and Deep Learning
Artificial intelligence (AI), and its enhancing mathematical systems for estimation and classification, is one of the fields of computer science that was conceived around the beginning of the 1940s. AI is primarily centered on mathematical models that mimic the functioning of the human brain. ML is a branch of AI where a system learns from large amounts of data samples and provide the conclusive result for classification and regression [14-16]. As the size of the data set increases learning improves, and it becomes possible via ML to estimate unknowns and to predict outputs. As formally defined by Tom Mitchell: “A computer program is said to learn from experience E with respect to some class of tasks T and performance measure P, if its performance at tasks in T, as measured by P, improves with experience E” [17]. ML has been seen as the new field of research for biomedical studies and provides a variety of applications in various sub- domains like cancer detection and monitoring [18]. ML algorithms are completely dynamic in nature and always try to improve as more data are added to the dataset. Mostly ML algorithms are represented as mathematical models, where data samples map to observed variables termed as features, and as variables outcome termed as labels [14-19]. The optimization of algorithms through a particular process termed as training employs the training set (of available data from previous measurements) and as output, it predicts the labels by extracting and analyzing the exact features, even with newly added data samples.
Classification of Machine Learning Techniques
ML techniques can be classified based on label type and feature type. Label type techniques are mostly categorized into three: (i) supervised, (ii) unsupervised, and, (iii) reinforcement learning. Based on the type of features the classification is mostly as (i) handcrafted, and;(ii) non-handcrafted feature-based techniques.
Supervised Learning: Supervised learning applies to exact data sets that are labeled by working researchers of their particular research areas and by industry professionals. Feature engineering algorithms are used to train and reduce the quantified prediction error rates, that is, the difference between the predicted labels and the known labels. In general, types of algorithms that are employed are: linear and logistic regression, Naive Baye’s classification [19], support vector machines (SVMs) [14], and random forests [20]. The histopathology based classification, for example, is one of the supervised learning applications where pathological images are labeled by the expert for cancer versus non-cancer and for different Gleason grades [21-23].
Unsupervised Learning
Unsupervised learning applies to datasets where the algorithm separates into different classes, depending on the input features and training data, which is not explicitly labeled. In general, types of algorithms employed include k-means clustering [19] which involves finding groups or clusters of provided data, principal component analysis (PCA) [24], and, autoencoders [25]. The unsupervised learning-based algorithms are applied to recognize patterns of immunohistochemical staining when stained for histone modifications in tissue samples. The study inferred that different patterns lead to variations in the amount of risk of the repetitiveness of cancer and it is almost independent of applying tumor stage or PSA type of clinical parameters [26].
Reinforcement Learning
Reinforcement learning is applied to datasets where the algorithm and its function are conclusive. The reinforcement algorithm invokes an assistant that acts to predict the features for further prospective steps. It always depends on the present and past features of datasets. The assistant ultimately learns from selecting the individual and takes action at each stage to maximize the expectation.
Feature-Based ML Techniques
These techniques utilize handcrafted and non- handcrafted features for ML. Handcrafted-feature based implementation is associated with the derivation of infinite numbers of exact features. As mentioned earlier, these features are provided by biomedical experts who are looking for them in their diagnostic or decision process. Selective features are mostly based on subject matter experts who possess a vast knowledge of the corresponding topic. Dataset designed confine to various features captured from glands and tarnish histopathology slide’s nuclei per unit area feature and its corresponding shape and statistical properties. ML techniques associated with preprocessing step calibrates proper algorithms like edge or object detection for image processing are employed [27]. Non-handcrafted feature-based techniques associate the derivation of the raw data for learning. The algorithm learns and adapts to extract its features even without exact labeling and tries to minimize the prediction error. These methods scale with data, that is, as larger data sets are used for training, their performance improves and the resulting features may not necessarily be interpretative by humans [5-25-28].
The generated large amount of clinical laboratory reports and medical data are generally in the form of text, which is not properly structured, and is incomprehensible for the computer program, whereas image EP (expand EP) and genetic data are mostly logical to the machine so that the ML algorithms are easily implemented once it uses preprocessed data. The implementation of ML methodologies to data samples establish the basic segments. Captured data may subject an issue related to the quality of the data and after completing the pre- processing implementation of ML algorithms become more suitable. These biomedical captured data possibly have outliers, missing data, and noise due to duplicate data that degrade data quality. ML algorithms and their performance and analysis improve by improving data quality.
Figure 1 represents a summary of the work-flow of a biomedical imaging system that implements a machine learning and deep learning methodology. Study shows that different techniques are available for data preprocessing that focus on modifying the data for better fitting in a specific ML method [16].
Figure 1: Radiomic Workflow for Machine Learning & Deep Learning Approach for Biomedical Imaging.
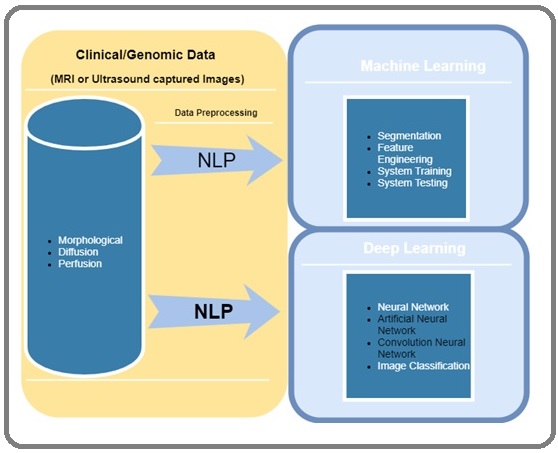
Technique implemented for data preprocessing includes feature selection, dimensionality reduction, and feature extraction. Dimensionality reduction improves preprocessing and implements when the number of features in the datasets present, the output results of ML algorithms perform better and significant performance improvement can be obtained when the dimensionality is lower [29]. Feature extraction comprises selecting a subset of all features that are sufficient to capture a significant amount of relevant information in a dataset.
Deep Learning
Deep learning is a branch of machine learning that primarily deals with algorithms derived from the functioning and structure of human brains- Artificial neural networks. ANN (Artificial neural network) generated a diversity of classification or pattern recognition problem-solving scenarios. Numerous hidden layers produce neural connections based on mathematical concepts that are generally used in ANN system. Researcher have shown that biomedical systems mostly represent nonlinear systems, thereby making ANNs a valuable computing tool for research in the field of biology. AI is being applied to various aspects of cancer for few decades [30]. With the ongoing research, the use of computational methods have only become more effective than before.
AI Applications in Cancer Imaging
AI-based deep learning algorithms have been implemented to identify complex patterns in medical and clinical images. They attempt to translate images and complement clinical decisions, thereby enabling meaningful decisions that are most times hard for humans. AI enhances the gathering of various data streams into dynamically integrated symptomatic systems. These include radiographic images studied in pathology, genomics and capturing electronic health reports, and social networks. The study of cross-sectional radiographic images reproduced by MRI and CT scanning is always challenging in recognizing complicated patterns. Whereas computers can potentially be trained efficiently to get results that can be produced rapidly. ML can be implemented for MRI datasets or digitally captured images. Figure 2 describes cancer detection phases for image processing. Low-level transformation methods are used to implement classification of images that are the initial stage of image analysis like segmentation and registration, and are mathematical formulated using statistical and biomechanical modeling and targeted to solve computer vision-based image processing.
Figure 2: Cancer Detection Stages by Image Processing Methodology.
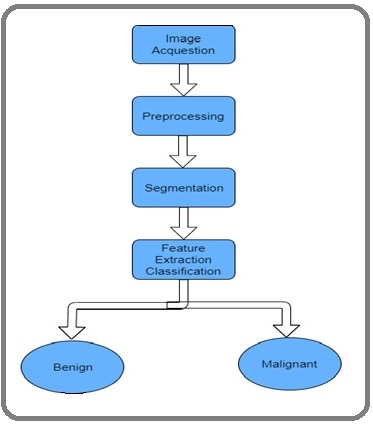
Higher-level transformation-based tasks have provided relevant info corresponding to prostate cancer detection, characterization, and grading. Cancer imaging based on AI implementation provides great applicability and flexibility and enhances three major biomedical works like sensing, classification, and treatment monitoring of tumors.
Computer-aided detection (CADe) is a term used for detection associated with finding objects in radiographs.
CADe has been used for companion assistant in recognizing hidden cancers in cases of low-quantity CT screening [31] and identifying brain tumor progression in MRI images with tremendous sensitivity during detection [32]. CADe have also aided in mammography right from spotting micro-calcification clusters to the indexing of the initial stage of breast cancer lump [33]. Recent studies have proved CADe to be efficient reducing some of the diagnostic constraints like inter-rater bias, irregular regenerative reports by biomedical professionals, time utilization as well as labor [34-35]. Application-based on AI adds high efficiency in the recreation of the nature of tumor productively with automated segmentation. Images of the entire body can potentially be interpreted by AI algorithms to perform tasks of segmentation. Its performance can be enhanced in the identification of organ structures which is mostly not detected by most personnel except for a pathological expert. The radiologic data are being used to train AI in the diagnosis of skeptical lesions and classify them as benign or malignant. Recent research works are mainly working on tumor extension and multi-nodality in breast MRI [36]. The newly computerized lesion depends on the volume-based analysis tools in contrast-upgraded magnetic resonance mammography (MRM) [37]. The advancement of genomics study from a data outlook maintains collaborative scope by adding AI-based imaging endeavor [38].
World Health Organization (WHO) and Response Evaluation Criteria in Solid Tumors (RECIST) principally work to identify difficulties in conventional physical tracking of tumors and resolve them. Various biomarkers are also being studied and implemented for cancer treatment in addition to their use as an alternative for continuous tracking of cancer. Investigation of circulating tumor DNA (ctDNA) discharged from tumor cells contributes toward the recent and dynamic state of art of work in the field of cancer and enhances tracking of disease evolution [39-42]. AI implemented unified treatment connecting molecular and pathological information with image-based searching that could aid in decision making. The various fields where AI can be implemented in cancer research are listed in Table 1.
Sl No. | Fields where AI can be implemented |
1 | Prediction of cancer in its early stages by analyzing CT scan images [100] |
2 | Identification of cancer during screening [45] |
3 | Differentiate lesions that are indolent versus aggressive lesion [101] |
4 | Differentiate benign and malignant lesions in medical images [63] |
5 | Evaluation of both clinical and molecular characterization of tumors via radiomics [102] |
6 | Prediction of mutational status in tumors via radiomics [54] |
7 | Identify prognostic phenotype of a tumor [103] |
8 | Predict clinical outcome in patients treated with immunotherapy [104] |
9 | Aid in subtype classification of tumors [70] |
10 | Predict recurrence-free survival rate [105] |
Applications of AI in Lung Cancer
Biomedical imaging and ML provided a new dimension to research. The initial stage of lung cancer detection is always important. ML added new features and possibilities to enhance lung cancer diagnosis and tracking treatment response. Various models are being designed to propagate the initial stage of detection and enable AI to meaningfully categorize lung nodules into two classes namely benign or malignant [43-45].
The National Lung Screening Trial (NLST) exhibited a 20% decrement in mortality rate in lung cancers in recent and old smokers obscurely with the use of low-dose CT (LDCT) for screening [46]. NLST exhibits a list of constraints that confide to distinguish the initial stages of lung cancer which can potentially be solved by the computational approach [46-49]. Till-date, there are no authenticated and verified approach setups to categorize whether nodules are malignant or benign. Classical biostatistics and ML methodology implement to discuss various obstruction in lung cancer screening. ML has shown multiple possibilities and newer techniques to recognize biomarkers to minimize imaging false-positive outcomes and more precisely categorize benign and malignant nodules. The majority of inclusive pulmonary nodules are unexpectedly detected posing a problem to cancer victims [50]. In a recent study, four quantitatively scored semantic features such as short-axis radius, contour, concavity, and texture were considered in an ML model to classify benign or malignant nodules in the lung cancer screening setting. The model classified the nodules with an accuracy of 74.3% [44].
Image-based biomarkers can be stored in the radiographs and featured into the elemental pathophysiology of a tumor. Clinical and biomedical implementation depends on size-based measurement and gives an appropriate estimation on prognostic factors such as survival and recurrence rates [51-54]. AI methods are being explored to quantify phenotypic characteristics of radiographic images based on the presence of specific mutations employing predefined algorithms and deep learning (a process termed as radiomics) [54]. Research works numerous cancer types like lung cancer have provided results with P less than 3.53 × 10−6 [54].
Applications of AI in Breast Cancer
A statistical report says, among the various cancers, breast cancer is the most frequently diagnosed cancer [55]. Breast cancer can be classified as a heterogeneous disease wherein there is a wide variation with respect to the size of tumors, prognosis, etiology as well as response to treatment. Recent advances in imaging, as well as computer systems, have resulted in a rapid rise in the potential use of AI for numerous amounts of tasks in the field of breast imaging. These AI applications are mostly applied for diagnosis and prediction treatment response and prognosis [56-57- 66-71-58-65].
Breast cancer screening is done using an imaging technique called CADe and CADx. Study shows a large amount of work has been done in this field in the last decades [71-73]. CADe is mostly applied to distinguish mammography translation and it has been part of regular biomedical applications since 1990 [72-73]. Various challenges present in the detection of cancer by radiologists include complex noise (incomplete visual search patterns, camouflaging normal anatomic background), fatigue, the estimation of the indirect complicated state of diseases, a huge number of image data and the quality of the image. CADe based implementation remains as a continuing research field in mammography to automatize the identification of breast lesions based on MRI, 3D ultrasound and tomosynthesis images by consolidating already defined algorithms and deep learning methodology [74-77]. CNN’s model is applied for the identification of mammograms [56] and studies show deep learning methodology [59] to provide great flexibility on CAD of breast lesions in ultrasound, MRI, and mammography [74-77].
Computer vision-based deep learning algorithms have frequently been applied in the past few years to identify the volume/density dimension in breast images and identify parenchyma arrangement, significant biomarkers for cancer risk estimation and finally to illustrate the treatment management. As the density of images goes high, it raises risk factors for breast cancer which could have an obscure effect in the identification of those lesions. Volumetric- based estimation of density are more likely applied [78-80]. In full-field digital mammography (FFDM), tissues are categorized into different classes based on differences in x-ray signal attenuation of fibroglandular and fat tissues. The other feature is variability in parenchymal patterns depending upon the spatial distribution of dense tissue which are image-based risk factors. Deep learning-based Texture looks into BRCA1/BRCA2 gene mutations and parenchymal patterns analyze the risk of breast cancer and chances of occurrence. Results achieved an AUC of relatively around 0.82 [71-81-82].
In recent years, biomarkers have improved and provided benefits along with the combination of information from detailed patient reports and datasets like biological cellular imaging and genomic data captured during symptomatic tasks and consecutive surgery. NCI’s TCGA Breast Phenotype Group collected interdisciplinary analysts for phenotypically investigating substantial breast tumors to achieve image-based information for molecular characteristics as well as gene expression profiles [83- 85].The MRI-genomic cooperative is being explored to the new dimension and provides a good generous genetic structure that standardizes the evolution of tumor phenotypes [66-83].
Research society has been actively working from the 1980s to promote ML techniques for CADx. The purpose is to perform the work of classifying benign and malignant breast lesions [73]. CADx with AI implemented computerized tumor classification and basic level representation as done by a radiologist expert. The AI-based software system can be used to characterize the skeptical lesion, predict prognosis of cancer and also provide a patient tracking system to the specialist. AI-based software systems are being extensively used in breast cancer and captured image data are being successfully classified based on tumor size, kinetics, texture, shape and morphology [86].
Application of AI in CNS Tumor
CNS tumors occurrence present itself with a large spectrum in the field of pathology and are possibly more diverse with respect to any other tumors in the human body. This wide range of diagnoses demands a very unique and accurate estimation of imaging modalities. One of the most important biomarkers that aid in determining the prognosis in CNS tumors is Isocitrate Dehydrogenase (IDH). The changes in the presence of IDH mutation can be effectively recognized using machine learning methods including deep CNNs trained on conventional MR images [87-88]. Technically similar kind of work has been already done on other brain tumors. The study results demonstrated that algorithms trained to extract radiomics features from conventional MRI can generate predictive models for pituitary adenoma subtypes and pediatric brain tumors. Various challenges arise when distinguishing between different tumor types. One of the major challenges faced in the diagnosis of CNS tumors is differentiating between primary CNS lymphoma and glioblastoma due to their similarity in imaging phenotypes. Results have shown radiomics models using image texture-based features to boost the differences between glioblastoma and primary CNS lymphoma [87-89]. Interestingly, a similar diagnostic dilemma often arises when evaluating histopathology slides of these same two different disease processes [90]. Recent research implemented AI in brain tumors focusing on efficiently categorizing biological and histopathologic subclass of brain tumors [87-91]. AI-based system demanding new models to accurately classify tumors requires dataset testing and training of a large number of corresponding data. Thereby AI would aid in providing better treatment quality with increasing accuracy of discrimination among multiple tumors [92].
Treatment of tumors is decided upon by the accurate classification of tumor subclasses. MR imaging is very useful in the process of defining CNS neoplasms. These tumors may reveal with different classes of contrast improvement and possibly be linked with hemorrhage and peritumoral edema or may blur in the limit from adjacent bone, blood vessels, fat, or surgical packing materials. The automatic identification of CNS tumors is expected to develop strong density-based algorithms to describe tumor as well as link them with the microenvironment that play a major role. Recent studies and methodologies implemented on automatic and semiautomatic detection of CNS tumors majorly applied to conventional MR imaging, ultrasound and PET images [93]. Research has been conducted where models are being created for applications such as treatment planning stereotactic radiosurgery [94], volume-based detection of residual tumor after surgery and tracking tumor growth over time [93]. Algorithms that automatically detect a tumor in the evaluation of patients having numerous intracranial lesions could be of great advantage to monitor metastases, growth rate and response to treatment over time. In the case of lesions in the skull- base that are mostly irregularly shaped extending across extracranial and intracranial compartments,
AI could help in automatic volumetric
reconstruction and detecting sensitive variations in growth which are often missed out by a normal observer. Spatial classification of heterogeneous tissues present in both tumor lesions and treatment-related changes remains to be a challenge. However, via the machine-learning approach, we can combine multiple imaging features thereby improve the ability to create a tissue classifier that would not only be accurate but also takes the heterogeneity of treated tumors into account. One of the examples include differentiating radionecrosis from recurrent brain tumors using conventional
MRI based Texture features extraction [95-96]. MRI sequences based on susceptibility-weighted and perfusion- weighted can also be integrated to differentiate between recurrence and radionecrosis in patients with high-grade gliomas
Another area where ML is being explored in the discovering of image biomarkers. The research on image biomarkers concentrates on finding associations between radiological features and histologic features. One such example includes the use of supervised machine learning in predicting the status of MGMT (methylation of the O(6)-methylguanine methyltransferase) in preoperative glioblastoma multiforme tumors, where the model exhibited a maximum area under the receiver-operating characteristic (ROC) curve of 0.85 (95% CI: 0.78- 0.91). Radiomic based system is also being designed by implementing traditional and diffusion MR imaging characteristics to identify the life span of survivors [97].
Applications of AI in Prostate Cancer
The clinical non-uniformity of prostate cancer with reference to tumor size (very less to extremely destructive tumors), high recurrence and varying mortality rate from patient to patient poses a lot of challenges in itself. An ML-based supervised technique is frequently applied to imaging modalities like US imaging to find skeptical lesions and provide full extent biological advantage in cancer studies. Deep learning-based application in prostate cancer would be beneficial for treatment and generating high-performance results.
Multi-parametric magnetic resonance imaging (mpMRI) are capable of portraying soft-tissue contrast for the identification of doubtful prostate lesions and provide insight into tissue properties. Study shows mpMRI is a promising imaging technique for prostate cancer due to its potential to find lesions and provide surgical features. Identification and classification of prostate tumors based on AI models provided flexibility with the advancement in CADe and CADx systems [98]. In partnership with PI-RADS, CAD systems could possibly improve the feasibility and treatment accuracy of mpMRI [99]. Initial work on mpMRI based CADx systems targeted on supervised learning model, adding feature extraction and trivial classification. The report stated that feature extraction plays a major role in enhancing system outcomes depending upon CAD. CNN’s added the full extension of work and convey good performance report in prostate cancer identification and treatment. A different feature of CNN algorithms such as an auto windowing are added for better MRI image classification and normalization with the addition of mpMRI images [99].
Limitations and Future prospects of AI in cancer
AI is continuing to prove its potential and efficacy in various stages of disease confronting such as early detection, treatment planning and prediction of future outcomes. Despite the increased advancements being made in AI and its applications in oncology, there are numerous limitations and setbacks that needs to be addressed. Few of them include issues with data access, generalizability, developing real-world applications, interpretation issues, ‘black box’ problem, and challenges pertaining to education and expertise in the field. Although various literature evidences have proved AI to be efficient in diagnosing and outcome prediction of various cancers, the generalizability of the said AI application needs to be validated, as most studies would be confined to a particular disease type in a specific population, with data being obtained from a particular institution/repository.
Efforts needs to be made in terms of promoting medical data sharing among institutes and carrying out multiple external validations. Numerous attempts are being carried out in developing real world applications, however, AI training is a data-hungry method requiring a multi-faceted approach from all the institutions worldwide. Apart from data draughts arising from patient privacy issues and dearth of data-sharing facilities in institutions, obtaining a complete data with the required quality is yet another obstacle. Training of AI in diagnosing or prediction of a specific disease with proper data from various populations would strengthen the AI’s ability to perform with accuracy, irrespective of where the application would be used.
One of the other major challenges that’s being currently faced while using AI in medical domain, is trying to interpret as to how AI model came up with the solution. This limitation in the ability to precisely understand the logic behind these algorithms is termed as the “black box” problem. Various methods such as saliency maps, sensitivity analysis, feature visualization and class activation mapping are being utilized to tackle these issues. Further research is needed to decode and extract human understandable explanations from these AI algorithms. These explanations may pave way to develop newer and more efficient methods to understanding disease process, diagnosis and prediction of cancer.
In conclusions, the increasing incidence and mortality of cancer necessitate the need for more medical and technological advancements which would aid in early detection and better treatment. Advancements in Machine learning and artificial intelligence have reached a point where they are being incorporated in most of the fields in science including medicine. On the basis of wide research being done, AI is proving itself to be a very reliable adjunct to medical professionals and promises to significantly improve detection and therapeutic methods. However, more interdisciplinary research is required to generalize the clinical application of AI, machine learning and deep learning in all cancer types as well as in different fields of oncology. Such research should also aim at overcoming the challenges being faced and collectively aid in benefiting the patients and enhance better clinical outcomes.
Acknowledgements
None
Abbreviations
ML, Machine Learning; DL, Deep learning; CNN, Convolutional Neural Networks; AI, Artificial intelligence; SVM, Support Vector Machine; PCA, Principal Component Analysis; CADe, Computer-aided Detection; ctDNA, Circulating tumor DNA; NLST, National Lung Screening Trial; FFDM, Full-field Digital Mammography; ROC, Receiver-operating Characteristic; mpMRI, Multi-parametric Magnetic Resonance Imaging
Authors’ contributions
All authors contributed equally
Availability of data and material
The reviews was based on published articles
References
- World health statistics 2018: monitoring health for the SDGs, sustainable development goals OMS . 2018.
- Global Cancer Incidence and Mortality Rates and Trends--An Update Torre L. A., Siegel R. L., Ward E. M., Jemal A.. Cancer Epidemiology Biomarkers & Prevention.2015;25(1). CrossRef
- Comparison of breast mammography, sonography and physical examination for screening women at high risk of breast cancer in taiwan Hou Ming-Feng, Chuang Hung-Yi, Ou-Yang Fu, Wang Chen-Ya, Huang Chyi-Lie, Fan Hui-Mei, Chuang Chieh-Han, Wang Jaw-Yuan, Hsieh Jan-Singh, Liu Gin-Chung, Huang Tsung-Jen. Ultrasound in Medicine & Biology.2002;28(4). CrossRef
- Deep learning for image-based cancer detection and diagnosis − A survey Hu Zilong, Tang Jinshan, Wang Ziming, Zhang Kai, Zhang Ling, Sun Qingling. Pattern Recognition.2018;83. CrossRef
- Deep learning LeCun Yann, Bengio Yoshua, Hinton Geoffrey. Nature.2015;521(7553). CrossRef
- Deep Learning is Robust to Massive Label Noise Rolnick D, Veit A, Belongie S, et al . .
- Deep learning for multi-task medical image segmentation in multiple modalities. In: Lecture Notes in Computer Science (including subseries Lecture Notes in Artificial Intelligence and Lecture Notes in Bioinformatics) Moeskops P, Wolterink JM, van der Velden BHM, et al . Springer Verlag.2016;:478-486.
- Breast Cancer Detection Using Support Vector Machine Technique Applied on Extracted Electromagnetic Waves Al Sharkawy M, Sharkas M, Ragab D. 2012.
- FPGA-Based accelerators of deep learning networks for learning and classification: A review Shawahna A, Sait SM, El-Maleh A. IEEE Access.2019;7:7823-7859.
- Deep Learning in Medical Image Analysis Shen Dinggang, Wu Guorong, Suk Heung-Il. Annual Review of Biomedical Engineering.2017;19(1). CrossRef
- Overview of deep learning in medical imaging Suzuki Kenji. Radiological Physics and Technology.2017;10(3). CrossRef
- Emerging Intraoperative Imaging Modalities to Improve Surgical Precision Alam Israt S., Steinberg Idan, Vermesh Ophir, van den Berg Nynke S., Rosenthal Eben L., van Dam Gooitzen M., Ntziachristos Vasilis, Gambhir Sanjiv S., Hernot Sophie, Rogalla Stephan. Molecular Imaging and Biology.2018;20(5). CrossRef
- Deep learning for computational biology Angermueller Christof, Pärnamaa Tanel, Parts Leopold, Stegle Oliver. Molecular Systems Biology.2016;12(7). CrossRef
- Pattern Recoginiton and Machine Learning. Springer-Verlag New York Bishop CM. 2006.
- The Discipline of Machine Learning. Pittsburgh, PA Mitchell TM. 2006.
- Data mining : practical machine learning tools and techniques Witten IH, Frank E, Hall MA. Morgan Kaufmann.2011.
- Machine Learning. 1st ed. New York: McGraw-Hill Education Mitchell TM. 1997.
- Introduction to Computational Intelligence Techniques and Areas of Their Applications in Medicine. In: Agah A (ed) Medical Applications of Artificial Intelligence Niknejad A, Petrovic D. CRC Press.2013;:51-70.
- ‘Pattern Classification (2nd Edition)’. 2nd ed. New York: Wiley-Interscience Duda R, Hart P, Stork D. 2001.
- Random forests Breiman L. Mach Learn .2001;45:5-32.
- Computer-aided gleason grading of prostate cancer histopathological images using texton forests Khurd Parmeshwar, Bahlmann Claus, Maday Peter, Kamen Ali, Gibbs-Strauss Summer, Genega Elizabeth M., Frangioni John V.. 2010 IEEE International Symposium on Biomedical Imaging: From Nano to Macro.2010. CrossRef
- Cascaded discrimination of normal, abnormal, and confounder classes in histopathology: Gleason grading of prostate cancer Doyle Scott, Feldman Michael D, Shih Natalie, Tomaszewski John, Madabhushi Anant. BMC Bioinformatics.2012;13(1). CrossRef
- Prostate Histopathology: Learning Tissue Component Histograms for Cancer Detection and Classification Gorelick Lena, Veksler Olga, Gaed Mena, Gomez Jose A., Moussa Madeleine, Bauman Glenn, Fenster Aaron, Ward Aaron D.. IEEE Transactions on Medical Imaging.2013;32(10). CrossRef
- Encyclopedia of Statistics in Behavioral Science. Hoboken, NJ: John Wiley & Sons, 2005. Epub ahead of print 2005 Everitt BS, Howell DC. .
- Deep Learning. MIT Press Goodfellow I, Bengio Y, Courville A. 2016.
- Global histone modification patterns predict risk of prostate cancer recurrence Seligson David B., Horvath Steve, Shi Tao, Yu Hong, Tze Sheila, Grunstein Michael, Kurdistani Siavash K.. Nature.2005;435(7046). CrossRef
- The Image Processing Handbook. CRC Press, 2016. Epub ahead of print Russ JC. 2016.
- Christopher M. Neural Networks for Pattern Recognition. New York: Oxford University Press, Inc Bishop . 1995.
- Steinbach M, Kumar V. Introduction to Data Mining. 1st ed Tan P-N. Pearson.2006.
- Applications of machine learning in cancer prediction and prognosis Cruz JA, Wishart DS. Cancer Informatics.2006;2:59-77.
- Low-Dose CT Screening for Lung Cancer: Computer-aided Detection of Missed Lung Cancers Liang Mingzhu, Tang Wei, Xu Dong Ming, Jirapatnakul Artit C., Reeves Anthony P., Henschke Claudia I., Yankelevitz David. Radiology.2016;281(1). CrossRef
- Computer-aided detection of metastatic brain tumors using automated three-dimensional template matching Ambrosini Robert D., Wang Peng, O'Dell Walter G.. Journal of Magnetic Resonance Imaging.2009;31(1). CrossRef
- Computer-aided detection and classification of microcalcifications in mammograms: a survey Cheng H.D., Cai Xiaopeng, Chen Xiaowei, Hu Liming, Lou Xueling. Pattern Recognition.2003;36(12). CrossRef
- Validation of image segmentation by estimating rater bias and variance Warfield Simon K, Zou Kelly H, Wells William M. Philosophical Transactions of the Royal Society A: Mathematical, Physical and Engineering Sciences.2008;366(1874). CrossRef
- Heterogeneity in head and neck IMRT target design and clinical practice Hong Theodore S., Tomé Wolfgang A., Harari Paul M.. Radiotherapy and Oncology.2012;103(1). CrossRef
- The Eighth Edition AJCC Cancer Staging Manual: Continuing to build a bridge from a population-based to a more “personalized” approach to cancer staging Amin Mahul B., Greene Frederick L., Edge Stephen B., Compton Carolyn C., Gershenwald Jeffrey E., Brookland Robert K., Meyer Laura, Gress Donna M., Byrd David R., Winchester David P.. CA: A Cancer Journal for Clinicians.2017;67(2). CrossRef
- Computer-aided detection (CAD) system for breast MRI in assessment of local tumor extent, nodal status, and multifocality of invasive breast cancers: preliminary study Song Sung, Seo Bo, Cho Kyu, Woo Ok, Son Gil, Kim Chulhan, Cho Sung, Kwon Soon-Sun. Cancer Imaging.2015;15(1). CrossRef
- Size assessment of breast lesions by means of a computer-aided detection (CAD) system for magnetic resonance mammography Levrini G., Sghedoni R., Mori C., Botti A., Vacondio R., Nitrosi A., Iori M., Nicoli F.. La radiologia medica.2011;116(7). CrossRef
- Liquid biopsies come of age: towards implementation of circulating tumour DNA Wan Jonathan C. M., Massie Charles, Garcia-Corbacho Javier, Mouliere Florent, Brenton James D., Caldas Carlos, Pacey Simon, Baird Richard, Rosenfeld Nitzan. Nature Reviews Cancer.2017;17(4). CrossRef
- Phylogenetic ctDNA analysis depicts early-stage lung cancer evolution Abbosh Christopher, Birkbak Nicolai J., Wilson Gareth A., Jamal-Hanjani Mariam, Constantin Tudor, Salari Raheleh, Le Quesne John, Moore David A., Veeriah Selvaraju, Rosenthal Rachel, Marafioti Teresa, Kirkizlar Eser, Watkins Thomas B. K., McGranahan Nicholas, Ward Sophia, Martinson Luke, Riley Joan, Fraioli Francesco, Al Bakir Maise, Grönroos Eva, Zambrana Francisco, Endozo Raymondo, Bi Wenya Linda, Fennessy Fiona M., Sponer Nicole, Johnson Diana, Laycock Joanne, Shafi Seema, Czyzewska-Khan Justyna, Rowan Andrew, Chambers Tim, Matthews Nik, Turajlic Samra, Hiley Crispin, Lee Siow Ming, Forster Martin D., Ahmad Tanya, Falzon Mary, Borg Elaine, Lawrence David, Hayward Martin, Kolvekar Shyam, Panagiotopoulos Nikolaos, Janes Sam M., Thakrar Ricky, Ahmed Asia, Blackhall Fiona, Summers Yvonne, Hafez Dina, Naik Ashwini, Ganguly Apratim, Kareht Stephanie, Shah Rajesh, Joseph Leena, Marie Quinn Anne, Crosbie Phil A., Naidu Babu, Middleton Gary, Langman Gerald, Trotter Simon, Nicolson Marianne, Remmen Hardy, Kerr Keith, Chetty Mahendran, Gomersall Lesley, Fennell Dean A., Nakas Apostolos, Rathinam Sridhar, Anand Girija, Khan Sajid, Russell Peter, Ezhil Veni, Ismail Babikir, Irvin-Sellers Melanie, Prakash Vineet, Lester Jason F., Kornaszewska Malgorzata, Attanoos Richard, Adams Haydn, Davies Helen, Oukrif Dahmane, Akarca Ayse U., Hartley John A., Lowe Helen L., Lock Sara, Iles Natasha, Bell Harriet, Ngai Yenting, Elgar Greg, Szallasi Zoltan, Schwarz Roland F., Herrero Javier, Stewart Aengus, Quezada Sergio A., Peggs Karl S., Van Loo Peter, Dive Caroline, Lin C. Jimmy, Rabinowitz Matthew, Aerts Hugo J. W. L., Hackshaw Allan, Shaw Jacqui A., Zimmermann Bernhard G., Swanton Charles. Nature.2017;545(7655). CrossRef
- Direct detection of early-stage cancers using circulating tumor DNA Phallen Jillian, Sausen Mark, Adleff Vilmos, Leal Alessandro, Hruban Carolyn, White James, Anagnostou Valsamo, Fiksel Jacob, Cristiano Stephen, Papp Eniko, Speir Savannah, Reinert Thomas, Orntoft Mai-Britt Worm, Woodward Brian D., Murphy Derek, Parpart-Li Sonya, Riley David, Nesselbush Monica, Sengamalay Naomi, Georgiadis Andrew, Li Qing Kay, Madsen Mogens Rørbæk, Mortensen Frank Viborg, Huiskens Joost, Punt Cornelis, van Grieken Nicole, Fijneman Remond, Meijer Gerrit, Husain Hatim, Scharpf Robert B., Diaz Luis A., Jones Siân, Angiuoli Sam, Ørntoft Torben, Nielsen Hans Jørgen, Andersen Claus Lindbjerg, Velculescu Victor E.. Science Translational Medicine.2017;9(403). CrossRef
- Detection and localization of surgically resectable cancers with a multi-analyte blood test Cohen Joshua D., Li Lu, Wang Yuxuan, Thoburn Christopher, Afsari Bahman, Danilova Ludmila, Douville Christopher, Javed Ammar A., Wong Fay, Mattox Austin, Hruban Ralph H., Wolfgang Christopher L., Goggins Michael G., Dal Molin Marco, Wang Tian-Li, Roden Richard, Klein Alison P., Ptak Janine, Dobbyn Lisa, Schaefer Joy, Silliman Natalie, Popoli Maria, Vogelstein Joshua T., Browne James D., Schoen Robert E., Brand Randall E., Tie Jeanne, Gibbs Peter, Wong Hui-Li, Mansfield Aaron S., Jen Jin, Hanash Samir M., Falconi Massimo, Allen Peter J., Zhou Shibin, Bettegowda Chetan, Diaz Luis A., Tomasetti Cristian, Kinzler Kenneth W., Vogelstein Bert, Lennon Anne Marie, Papadopoulos Nickolas. Science.2018;359(6378). CrossRef
- Predicting Malignant Nodules from Screening CT Scans Hawkins Samuel, Wang Hua, Liu Ying, Garcia Alberto, Stringfield Olya, Krewer Henry, Li Qian, Cherezov Dmitry, Gatenby Robert A., Balagurunathan Yoganand, Goldgof Dmitry, Schabath Matthew B., Hall Lawrence, Gillies Robert J.. Journal of Thoracic Oncology.2016;11(12). CrossRef
- Radiological Image Traits Predictive of Cancer Status in Pulmonary Nodules Liu Ying, Balagurunathan Yoganand, Atwater Thomas, Antic Sanja, Li Qian, Walker Ronald C., Smith Gary T., Massion Pierre P., Schabath Matthew B., Gillies Robert J.. Clinical Cancer Research.2016;23(6). CrossRef
- Towards automatic pulmonary nodule management in lung cancer screening with deep learning Ciompi Francesco, Chung Kaman, van Riel Sarah J., Setio Arnaud Arindra Adiyoso, Gerke Paul K., Jacobs Colin, Scholten Ernst Th., Schaefer-Prokop Cornelia, Wille Mathilde M. W., Marchianò Alfonso, Pastorino Ugo, Prokop Mathias, van Ginneken Bram. Scientific Reports.2017;7(1). CrossRef
- Reduced Lung-Cancer Mortality with Low-Dose Computed Tomographic Screening New England Journal of Medicine.2011;365(5). CrossRef
- Overdiagnosis in Low-Dose Computed Tomography Screening for Lung Cancer Patz Edward F., Pinsky Paul, Gatsonis Constantine, Sicks JoRean D., Kramer Barnett S., Tammemägi Martin C., Chiles Caroline, Black William C., Aberle Denise R.. JAMA Internal Medicine.2014;174(2). CrossRef
- Screening for lung cancer Manser Renée, Lethaby Anne, Irving Louis B, Stone Christine, Byrnes Graham, Abramson Michael J, Campbell Don. Cochrane Database of Systematic Reviews.2013. CrossRef
- Indolent Potentially Inconsequential Lung Cancers in the Pittsburgh Lung Screening Study (PLuSS) Thalanayar Prashanth M, Altintas Nejat, Weissfeld Joel L, Fuhrman Carl R, Wilson David O. Annals of the American Thoracic Society.2015. CrossRef
- Incidental pulmonary nodules in emergent coronary CT angiography for suspected acute coronary syndrome: Impact of revised 2017 Fleischner Society Guidelines Scholtz Jan-Erik, Lu Michael T., Hedgire Sandeep, Meyersohn Nina M., Oliveira George R., Prabhakar Anand M., Gupta Rajiv, Kalra Mannudeep K., Shepard Jo-Anne O., Hoffmann Udo, Ghoshhajra Brian B.. Journal of Cardiovascular Computed Tomography.2018;12(1). CrossRef
- Radial gradient and radial deviation radiomic features from pre-surgical CT scans are associated with survival among lung adenocarcinoma patients Tunali Ilke, Stringfield Olya, Guvenis Albert, Wang Hua, Liu Ying, Balagurunathan Yoganand, Lambin Philippe, Gillies Robert J., Schabath Matthew B.. Oncotarget.2017;8(56). CrossRef
- CT imaging features associated with recurrence in non-small cell lung cancer patients after stereotactic body radiotherapy Li Qian, Kim Jongphil, Balagurunathan Yoganand, Qi Jin, Liu Ying, Latifi Kujtim, Moros Eduardo G., Schabath Matthew B., Ye Zhaoxiang, Gillies Robert J., Dilling Thomas J.. Radiation Oncology.2017;12(1). CrossRef
- Exploratory Study to Identify Radiomics Classifiers for Lung Cancer Histology Wu Weimiao, Parmar Chintan, Grossmann Patrick, Quackenbush John, Lambin Philippe, Bussink Johan, Mak Raymond, Aerts Hugo J. W. L.. Frontiers in Oncology.2016;6. CrossRef
- Somatic Mutations Drive Distinct Imaging Phenotypes in Lung Cancer Rios Velazquez Emmanuel, Parmar Chintan, Liu Ying, Coroller Thibaud P., Cruz Gisele, Stringfield Olya, Ye Zhaoxiang, Makrigiorgos Mike, Fennessy Fiona, Mak Raymond H., Gillies Robert, Quackenbush John, Aerts Hugo J.W.L.. Cancer Research.2017;77(14). CrossRef
- Global cancer statistics 2018: GLOBOCAN estimates of incidence and mortality worldwide for 36 cancers in 185 countries Bray Freddie, Ferlay Jacques, Soerjomataram Isabelle, Siegel Rebecca L., Torre Lindsey A., Jemal Ahmedin. CA: A Cancer Journal for Clinicians.2018;68(6). CrossRef
- Computerized detection of clustered microcalcifications in digital mammograms using a shift-invariant artificial neural network Zhang Wei, Doi Kunio, Giger Maryellen L., Wu Yuzheng, Nishikawa Robert M., Schmidt Robert A.. Medical Physics.1994;21(4). CrossRef
- Computer aided detection of masses in mammograms as decision support Karssemeijer N, Otten J D M, Rijken H, Holland R. The British Journal of Radiology.2006;79(special_issue_2). CrossRef
- Computerized mass detection for digital breast tomosynthesis directly from the projection images Reiser I., Nishikawa R. M., Giger M. L., Wu T., Rafferty E. A., Moore R., Kopans D. B.. Medical Physics.2006;33(2). CrossRef
- Computer-aided detection of clustered microcalcifications in digital breast tomosynthesis: A 3D approach Sahiner Berkman, Chan Heang-Ping, Hadjiiski Lubomir M., Helvie Mark A., Wei Jun, Zhou Chuan, Lu Yao. Medical Physics.2011;39(1). CrossRef
- Computerized analysis of breast lesions in three dimensions using dynamic magnetic-resonance imaging Gilhuijs Kenneth G. A., Giger Maryellen L., Bick Ulrich. Medical Physics.1998;25(9). CrossRef
- Improving breast cancer diagnosis with computer-aided diagnosis Jiang Yulei, Nishikawa Robert M., Schmidt Robert A., Metz Charles E., Giger Maryellen L., Doi Kunio. Academic Radiology.1999;6(1). CrossRef
- Volumetric texture analysis of breast lesions on contrast-enhanced magnetic resonance images Chen Weijie, Giger Maryellen L., Li Hui, Bick Ulrich, Newstead Gillian M.. Magnetic Resonance in Medicine.2007;58(3). CrossRef
- Cancerous Breast Lesions on Dynamic Contrast-enhanced MR Images: Computerized Characterization for Image-based Prognostic Markers Bhooshan Neha, Giger Maryellen L., Jansen Sanaz A., Li Hui, Lan Li, Newstead Gillian M.. Radiology.2010;254(3). CrossRef
- Exploring nonlinear feature space dimension reduction and data representation in breast CADx with Laplacian eigenmaps and t-SNE Jamieson Andrew R., Giger Maryellen L., Drukker Karen, Li Hui, Yuan Yading, Bhooshan Neha. Medical Physics.2009;37(1). CrossRef
- A novel and automatic mammographic texture resemblance marker is an independent risk factor for breast cancer Nielsen M., Karemore G., Loog M., Raundahl J., Karssemeijer N., Otten J.D.M., Karsdal M.A., Vachon C.M., Christiansen C.. Cancer Epidemiology.2011;35(4). CrossRef
- Digital mammographic tumor classification using transfer learning from deep convolutional neural networks Huynh Benjamin Q., Li Hui, Giger Maryellen L.. Journal of Medical Imaging.2016;3(3). CrossRef
- A deep feature fusion methodology for breast cancer diagnosis demonstrated on three imaging modality datasets Antropova Natalia, Huynh Benjamin Q., Giger Maryellen L.. Medical Physics.2017;44(10). CrossRef
- A bioinformatics analysis of the cell line nomenclature Sarntivijai Sirarat, Ade Alexander S., Athey Brian D., States David J.. Bioinformatics.2008;24(23). CrossRef
- Relationships between computer-extracted mammographic texture pattern features and BRCA1/2mutation status: a cross-sectional study Gierach Gretchen L, Li Hui, Loud Jennifer T, Greene Mark H, Chow Catherine K, Lan Li, Prindiville Sheila A, Eng-Wong Jennifer, Soballe Peter W, Giambartolomei Claudia, Mai Phuong L, Galbo Claudia E, Nichols Kathryn, Calzone Kathleen A, Olopade Olufunmilayo I, Gail Mitchell H, Giger Maryellen L. Breast Cancer Research.2014;16(4). CrossRef
- Quantitative MRI radiomics in the prediction of molecular classifications of breast cancer subtypes in the TCGA/TCIA data set Li Hui, Zhu Yitan, Burnside Elizabeth S, Huang Erich, Drukker Karen, Hoadley Katherine A, Fan Cheng, Conzen Suzanne D, Zuley Margarita, Net Jose M, Sutton Elizabeth, Whitman Gary J, Morris Elizabeth, Perou Charles M, Ji Yuan, Giger Maryellen L. npj Breast Cancer.2016;2(1). CrossRef
- Deep learning in breast cancer risk assessment: evaluation of convolutional neural networks on a clinical dataset of full-field digital mammograms Li Hui, Giger Maryellen L., Huynh Benjamin Q., Antropova Natalia O.. Journal of Medical Imaging.2017;4(04). CrossRef
- Computerized detection of breast cancer on automated breast ultrasound imaging of women with dense breasts Drukker Karen, Sennett Charlene A., Giger Maryellen L.. Medical Physics.2013;41(1). CrossRef
- Computer-aided Diagnosis in Radiology Lodwick Gwilym S.. Investigative Radiology.1966;1(1). CrossRef
- Computerized analysis of images in the detection and diagnosis of breast cancer Giger Maryellen L.. Seminars in Ultrasound, CT and MRI.2004;25(5). CrossRef
- Screening Mammography with Computer-aided Detection: Prospective Study of 12,860 Patients in a Community Breast Center Freer Timothy W., Ulissey Michael J.. Radiology.2001;220(3). CrossRef
- How Widely Is Computer-Aided Detection Used in Screening and Diagnostic Mammography? Rao Vijay M., Levin David C., Parker Laurence, Cavanaugh Barbara, Frangos Andrea J., Sunshine Jonathan H.. Journal of the American College of Radiology.2010;7(10). CrossRef
- Large scale deep learning for computer aided detection of mammographic lesions Kooi Thijs, Litjens Geert, van Ginneken Bram, Gubern-Mérida Albert, Sánchez Clara I., Mann Ritse, den Heeten Ard, Karssemeijer Nico. Medical Image Analysis.2017;35. CrossRef
- Classification of mass and normal breast tissue: a convolution neural network classifier with spatial domain and texture images Sahiner B., Heang-Ping Chan , Petrick N., Datong Wei , Helvie M.A., Adler D.D., Goodsitt M.M.. IEEE Transactions on Medical Imaging.1996;15(5). CrossRef
- Automated analysis of mammographic densities and breast carcinoma risk Byng Jeffrey W., Yaffe Martin J., Lockwood Gina A., Little Laurie E., Tritchler David L., Boyd Norman F.. Cancer.1997;80(1). CrossRef
- Computerized Analysis of Mammographic Parenchymal Patterns on a Large Clinical Dataset of Full-Field Digital Mammograms: Robustness Study with Two High-Risk Datasets Li Hui, Giger Maryellen L., Lan Li, Bancroft Brown Jeremy, MacMahon Aoife, Mussman Mary, Olopade Olufunmilayo I., Sennett Charlene. Journal of Digital Imaging.2012;25(5). CrossRef
- Computerized Analysis of Digitized Mammograms of BRCA1 and BRCA2 Gene Mutation Carriers Huo Zhimin, Giger Maryellen L., Olopade Olufunmilayo I., Wolverton Dulcy E., Weber Barbara L., Metz Charles E., Zhong Weiming, Cummings Shelly A.. Radiology.2002;225(2). CrossRef
- Parenchymal Texture Analysis in Digital Breast Tomosynthesis for Breast Cancer Risk Estimation Kontos Despina, Bakic Predrag R., Carton Ann-Katherine, Troxel Andrea B., Conant Emily F., Maidment Andrew D.A.. Academic Radiology.2009;16(3). CrossRef
- Computerized interpretation of breast MRI: Investigation of enhancement-variance dynamics Chen Weijie, Giger Maryellen L., Lan Li, Bick Ulrich. Medical Physics.2004;31(5). CrossRef
- MR Imaging Radiomics Signatures for Predicting the Risk of Breast Cancer Recurrence as Given by Research Versions of MammaPrint, Oncotype DX, and PAM50 Gene Assays Li Hui, Zhu Yitan, Burnside Elizabeth S., Drukker Karen, Hoadley Katherine A., Fan Cheng, Conzen Suzanne D., Whitman Gary J., Sutton Elizabeth J., Net Jose M., Ganott Marie, Huang Erich, Morris Elizabeth A., Perou Charles M., Ji Yuan, Giger Maryellen L.. Radiology.2016;281(2). CrossRef
- Defining a Radiomic Response Phenotype: A Pilot Study using targeted therapy in NSCLC Aerts Hugo J. W. L., Grossmann Patrick, Tan Yongqiang, Oxnard Geoffrey R., Rizvi Naiyer, Schwartz Lawrence H., Zhao Binsheng. Scientific Reports.2016;6(1). CrossRef
- Breast Image Analysis for Risk Assessment, Detection, Diagnosis, and Treatment of Cancer Giger Maryellen L., Karssemeijer Nico, Schnabel Julia A.. Annual Review of Biomedical Engineering.2013;15(1). CrossRef
- Residual Convolutional Neural Network for the Determination ofIDHStatus in Low- and High-Grade Gliomas from MR Imaging Chang Ken, Bai Harrison X., Zhou Hao, Su Chang, Bi Wenya Linda, Agbodza Ena, Kavouridis Vasileios K., Senders Joeky T., Boaro Alessandro, Beers Andrew, Zhang Biqi, Capellini Alexandra, Liao Weihua, Shen Qin, Li Xuejun, Xiao Bo, Cryan Jane, Ramkissoon Shakti, Ramkissoon Lori, Ligon Keith, Wen Patrick Y., Bindra Ranjit S., Woo John, Arnaout Omar, Gerstner Elizabeth R., Zhang Paul J., Rosen Bruce R., Yang Li, Huang Raymond Y., Kalpathy-Cramer Jayashree. Clinical Cancer Research.2017;24(5). CrossRef
- Multimodal MRI features predict isocitrate dehydrogenase genotype in high-grade gliomas Zhang Biqi, Chang Ken, Ramkissoon Shakti, Tanguturi Shyam, Bi Wenya Linda, Reardon David A., Ligon Keith L., Alexander Brian M., Wen Patrick Y., Huang Raymond Y.. Neuro-Oncology.2016;19(1). CrossRef
- Diffusion radiomics as a diagnostic model for atypical manifestation of primary central nervous system lymphoma: development and multicenter external validation Kang Daesung, Park Ji Eun, Kim Young-Hoon, Kim Jeong Hoon, Oh Joo Young, Kim Jungyoun, Kim Yikyung, Kim Sung Tae, Kim Ho Sung. Neuro-Oncology.2018;20(9). CrossRef
- Comparison between Glioblastoma and Primary Central Nervous System Lymphoma Using MR Image-based Texture Analysis Kunimatsu Akira, Kunimatsu Natsuko, Kamiya Kouhei, Watadani Takeyuki, Mori Harushi, Abe Osamu. Magnetic Resonance in Medical Sciences.2018;17(1). CrossRef
- CT-based radiomic signature predicts distant metastasis in lung adenocarcinoma Coroller Thibaud P., Grossmann Patrick, Hou Ying, Rios Velazquez Emmanuel, Leijenaar Ralph T.H., Hermann Gretchen, Lambin Philippe, Haibe-Kains Benjamin, Mak Raymond H., Aerts Hugo J.W.L.. Radiotherapy and Oncology.2015;114(3). CrossRef
- Large scale tissue histopathology image classification, segmentation, and visualization via deep convolutional activation features Xu Yan, Jia Zhipeng, Wang Liang-Bo, Ai Yuqing, Zhang Fang, Lai Maode, Chang Eric I-Chao. BMC Bioinformatics.2017;18(1). CrossRef
- An anatomic transcriptional atlas of human glioblastoma Puchalski Ralph B., Shah Nameeta, Miller Jeremy, Dalley Rachel, Nomura Steve R., Yoon Jae-Guen, Smith Kimberly A., Lankerovich Michael, Bertagnolli Darren, Bickley Kris, Boe Andrew F., Brouner Krissy, Butler Stephanie, Caldejon Shiella, Chapin Mike, Datta Suvro, Dee Nick, Desta Tsega, Dolbeare Tim, Dotson Nadezhda, Ebbert Amanda, Feng David, Feng Xu, Fisher Michael, Gee Garrett, Goldy Jeff, Gourley Lindsey, Gregor Benjamin W., Gu Guangyu, Hejazinia Nika, Hohmann John, Hothi Parvinder, Howard Robert, Joines Kevin, Kriedberg Ali, Kuan Leonard, Lau Chris, Lee Felix, Lee Hwahyung, Lemon Tracy, Long Fuhui, Mastan Naveed, Mott Erika, Murthy Chantal, Ngo Kiet, Olson Eric, Reding Melissa, Riley Zack, Rosen David, Sandman David, Shapovalova Nadiya, Slaughterbeck Clifford R., Sodt Andrew, Stockdale Graham, Szafer Aaron, Wakeman Wayne, Wohnoutka Paul E., White Steven J., Marsh Don, Rostomily Robert C., Ng Lydia, Dang Chinh, Jones Allan, Keogh Bart, Gittleman Haley R., Barnholtz-Sloan Jill S., Cimino Patrick J., Uppin Megha S., Keene C. Dirk, Farrokhi Farrokh R., Lathia Justin D., Berens Michael E., Iavarone Antonio, Bernard Amy, Lein Ed, Phillips John W., Rostad Steven W., Cobbs Charles, Hawrylycz Michael J., Foltz Greg D.. Science.2018;360(6389). CrossRef
- Automatic estimation of extent of resection and residual tumor volume of patients with glioblastoma Meier Raphael, Porz Nicole, Knecht Urspeter, Loosli Tina, Schucht Philippe, Beck Jürgen, Slotboom Johannes, Wiest Roland, Reyes Mauricio. Journal of Neurosurgery.2017;127(4). CrossRef
- A deep convolutional neural network-based automatic delineation strategy for multiple brain metastases stereotactic radiosurgery Liu Yan, Stojadinovic Strahinja, Hrycushko Brian, Wardak Zabi, Lau Steven, Lu Weiguo, Yan Yulong, Jiang Steve B., Zhen Xin, Timmerman Robert, Nedzi Lucien, Gu Xuejun. PLOS ONE.2017;12(10). CrossRef
- Support vector machine classification of brain metastasis and radiation necrosis based on texture analysis in MRI Larroza Andrés, Moratal David, Paredes-Sánchez Alexandra, Soria-Olivas Emilio, Chust María L., Arribas Leoncio A., Arana Estanislao. Journal of Magnetic Resonance Imaging.2015;42(5). CrossRef
- MRI features predict survival and molecular markers in diffuse lower-grade gliomas Zhou Hao, Vallières Martin, Bai Harrison X., Su Chang, Tang Haiyun, Oldridge Derek, Zhang Zishu, Xiao Bo, Liao Weihua, Tao Yongguang, Zhou Jianhua, Zhang Paul, Yang Li. Neuro-Oncology.2017;19(6). CrossRef
- Computer aided detection (CAD): an overview Castellino Ronald A.. Cancer Imaging.2005;5(1). CrossRef
- Computer Aided-Diagnosis of Prostate Cancer on Multiparametric MRI: A Technical Review of Current Research Wang Shijun, Burtt Karen, Turkbey Baris, Choyke Peter, Summers Ronald M.. BioMed Research International.2014;2014. CrossRef
- Early Detection and Prediction of Lung Cancer Survival using Neural Network Classifier Ada , Kaur R. .
- Noninvasive Computed Tomography–based Risk Stratification of Lung Adenocarcinomas in the National Lung Screening Trial Maldonado Fabien, Duan Fenghai, Raghunath Sushravya M., Rajagopalan Srinivasan, Karwoski Ronald A., Garg Kavita, Greco Erin, Nath Hrudaya, Robb Richard A., Bartholmai Brian J., Peikert Tobias. American Journal of Respiratory and Critical Care Medicine.2015;192(6). CrossRef
- Defining the biological basis of radiomic phenotypes in lung cancer Grossmann Patrick, Stringfield Olya, El-Hachem Nehme, Bui Marilyn M, Rios Velazquez Emmanuel, Parmar Chintan, Leijenaar Ralph TH, Haibe-Kains Benjamin, Lambin Philippe, Gillies Robert J, Aerts Hugo JWL. eLife.2017;6. CrossRef
- Decoding tumour phenotype by noninvasive imaging using a quantitative radiomics approach Aerts Hugo J. W. L., Velazquez Emmanuel Rios, Leijenaar Ralph T. H., Parmar Chintan, Grossmann Patrick, Carvalho Sara, Bussink Johan, Monshouwer René, Haibe-Kains Benjamin, Rietveld Derek, Hoebers Frank, Rietbergen Michelle M., Leemans C. René, Dekker Andre, Quackenbush John, Gillies Robert J., Lambin Philippe. Nature Communications.2014;5(1). CrossRef
- A radiomics approach to assess tumour-infiltrating CD8 cells and response to anti-PD-1 or anti-PD-L1 immunotherapy: an imaging biomarker, retrospective multicohort study Sun Roger, Limkin Elaine Johanna, Vakalopoulou Maria, Dercle Laurent, Champiat Stéphane, Han Shan Rong, Verlingue Loïc, Brandao David, Lancia Andrea, Ammari Samy, Hollebecque Antoine, Scoazec Jean-Yves, Marabelle Aurélien, Massard Christophe, Soria Jean-Charles, Robert Charlotte, Paragios Nikos, Deutsch Eric, Ferté Charles. The Lancet Oncology.2018;19(9). CrossRef
- Artificial intelligence for predicting recurrence-free probability of non-invasive high-grade urothelial bladder cell carcinoma Cai T, Conti G, Nesi G, et al . Oncol Rep.2007;18:959-964.
License

This work is licensed under a Creative Commons Attribution-NonCommercial 4.0 International License.
Copyright
© Asian Pacific Journal of Cancer Biology , 2020
Author Details